New Approach to Determining How Atoms Are Arranged in Materials
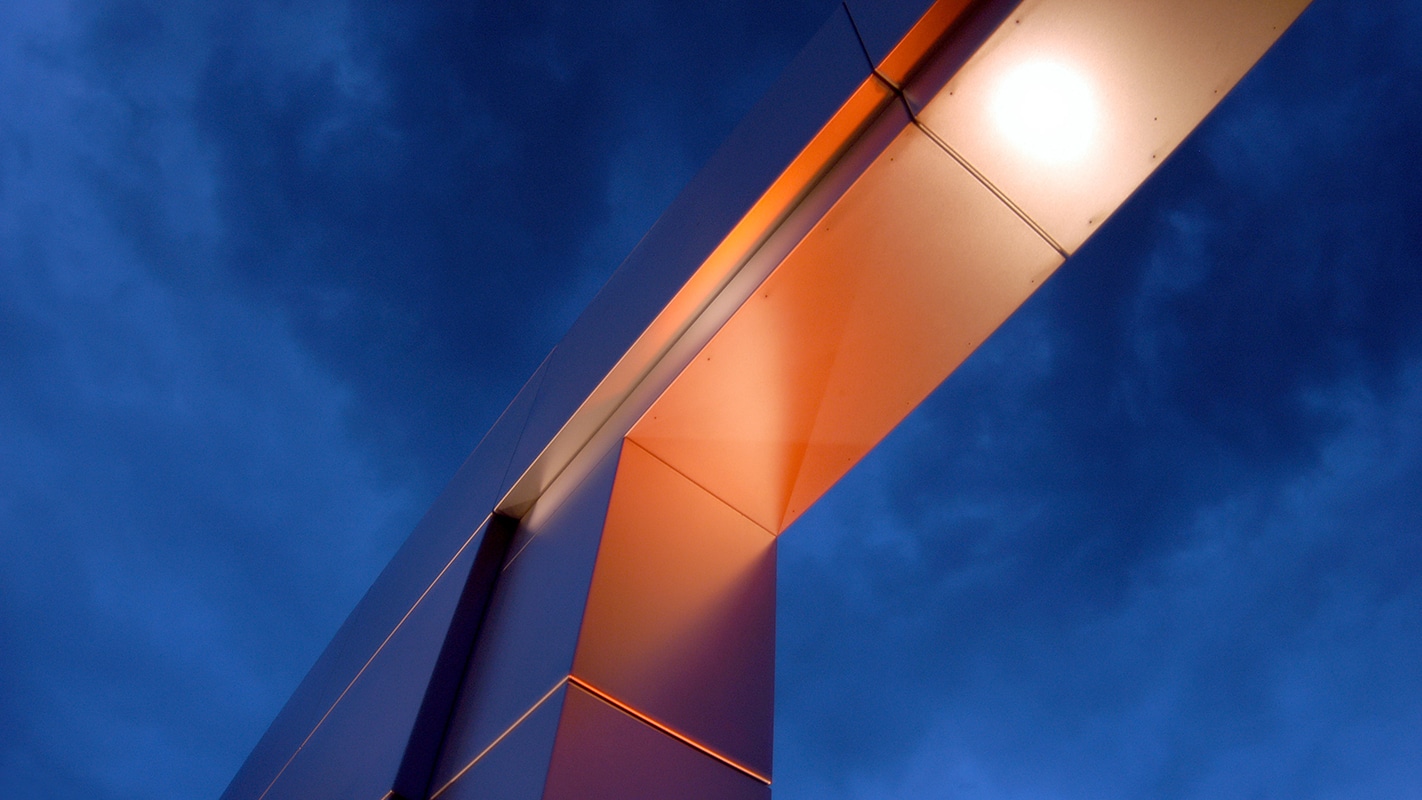
Researchers from North Carolina State University, the National Institute of Standards and Technology (NIST) and Oak Ridge National Laboratory (ORNL) have developed a novel approach to materials characterization, using Bayesian statistical methods to glean new insights into the structure of materials. The work should inform the development of new materials for use in a variety of applications.
“We want to understand the crystallographic structure of materials – such as where atoms are located in the matrix of a material – so that we have a basis for understanding how that structure affects a material’s performance,” says Jacob Jones, a professor of materials science and engineering at NC State and co-author of a paper on the work. “This is a fundamentally new advance that will help us develop new materials that can be used in everything from electronics and manufacturing to vehicles and nanotechnologies.”
The first step in understanding a material’s crystallographic structure is bombarding a sample of the material with electrons, photons or other subatomic particles, using technology such as the Spallation Neutron Source at ORNL or the Advanced Photon Source at Argonne National Laboratory. Researchers can then measure the angle and energy of these particles as they are scattered by the material.
Then things get really tricky.
Traditionally, the data from these scattering experiments has been analyzed using “least squares fitting” statistical techniques to infer a material’s crystallographic structure. But these techniques are limited; they can tell researchers what a material’s structure is likely to be – but they don’t fully describe the variability or uncertainty within the material’s structure, because they don’t describe the answers using probabilities.
“Least squares is a straightforward technique, but it doesn’t allow us to describe the inferred crystallographic structure in a way that answers the questions that the materials scientists want to ask,” says Alyson Wilson, a professor of statistics at NC State and co-author of the paper. “But we do have other techniques that can help address this challenge, and that’s what we’ve done with this research.”
In reality, the space between atoms isn’t constant – it’s not fixed throughout a sample. And the same is true for every aspect of a material’s structure.
“Understanding that variability, now possible with this new approach, allows us to characterize materials in a new, richer way,” Jones says.
This is where Bayesian statistics comes into play.
“For example, atoms vibrate,” Wilson says. “And the extent of the vibration is controlled by their temperature. Researchers want to know how those vibrations are influenced by temperature for any given material. And Bayesian tools can give us probabilities of these thermal displacements in a material.”
“This approach will allow us to analyze data from a wide variety of materials characterization techniques – all forms of spectroscopy, mass spectrometry, you name it – and more fully characterize all kinds of matter,” Jones says.
“Honestly, it’s very exciting,” adds Jones, who is also the director of NC State’s Analytical Instrumentation Facility, which houses many of these types of instruments.
“We also plan to use these techniques to combine data from different types of experiments, in order to offer even more insights into material structure,” Wilson says.
The paper, “Use of Bayesian Inference in Crystallographic Structure Refinement via Full Diffraction Profile Analysis,” is published in the Nature journal Scientific Reports. Lead authors of the paper are Chris Fancher, who is a postdoctoral researcher at NC State, and Zhen Han, a former Ph.D. student at NC State. Co-authors include Igor Levin of NIST; Katharine Page of ORNL; Brian Reich, an associate professor of statistics at NC State; and Ralph Smith, a Distinguished Professor of Mathematics at NC State. The work was done with support from the Kenan Institute for Engineering, Technology and Science at NC State, the Eastman Chemical Company-University Engagement Fund at NC State, the National Science Foundation under grant DMR-1445926, and the U.S. Department of Energy’s Office of Science under contract number DE-AC02-06CH11357.
-shipman-
Note to Editors: The study abstract follows.
“Use of Bayesian Inference in Crystallographic Structure Refinement via Full Diffraction Profile Analysis”
Authors: Chris M. Fancher, Zhen Han, Brian J. Reich, Ralph C. Smith, Alyson G. Wilson and Jacob L. Jones, North Carolina State University; Igor Levin, National Institute of Standards and Technology; Katharine Page, Oak Ridge National Laboratory
Published: Aug. 23, Scientific Reports
DOI: 10.1038/srep31625
Abstract: A Bayesian inference method for refining crystallographic structures is presented. The distribution of model parameters is stochastically sampled using Markov chain Monte Carlo. Posterior probability distributions are constructed for all model parameters to properly quantify uncertainty by appropriately modeling the heteroskedasticity and correlation of the error structure. The proposed method is demonstrated by analyzing a National Institute of Standards and Technology silicon standard reference material. The results obtained by Bayesian inference are compared with those determined by Rietveld refinement. Posterior probability distributions of model parameters provide both estimates and uncertainties. The new method better estimates the true uncertainties in the model as compared to the Rietveld method.
This post was originally published in NC State News.
- Categories: