Your Data, Your Treatment
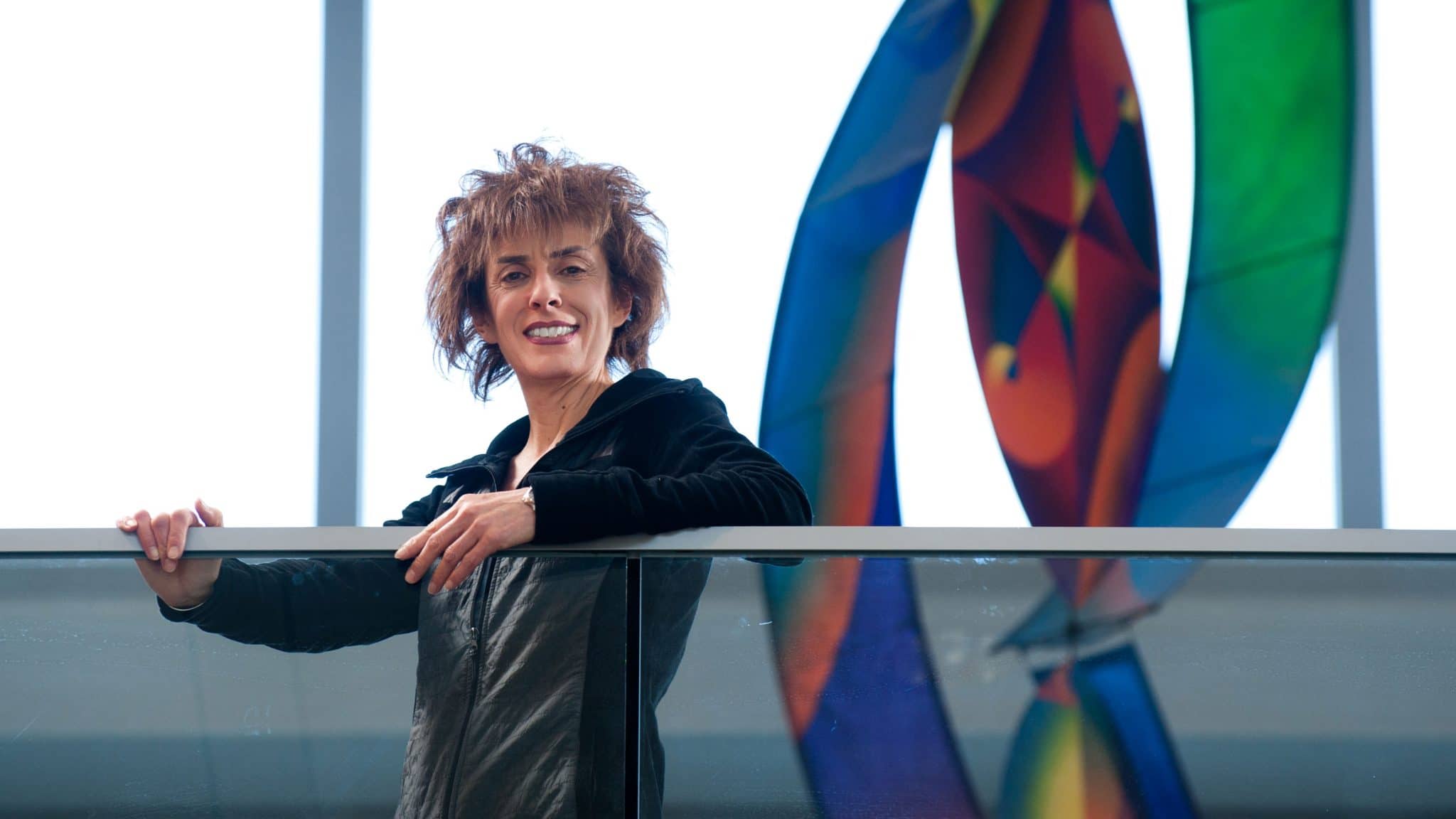
Viewpoint: Marie Davidian
The advent of the genomic era has made the prospect of “personalizing” treatment for individual patients more tantalizing than ever.
Media accounts abound of discoveries that tie a patient’s genetic and other characteristics to disease prognosis. Such revolutionary advancements could help determine not only which treatment a patient should receive, but spur the development of new treatments. Already, some cancer therapies specifically target patients with certain genetic profiles based on an understanding of the patients’ underlying biology.
Despite these successes, the goal of fully personalized medicine — selecting the best treatment based on an individual patient’s characteristics and history — remains mostly elusive. Why?
Determining the best treatment for an individual patient is often more complicated than identifying a single gene. Care that is truly personalized will require disentangling complex interactions between genetic, physiologic, environmental and other factors and a patient’s response to treatment. This involves making sense of reams of patient data to identify which characteristics, if any, are useful for determining treatment, and in what combination.
It’s thus no surprise that statistics — the discipline devoted to making sense of data, determining which data to collect, and designing studies to do so — is critical to the quest for personalized medicine.
The most popular approach is to identify “subgroups” of patients who share certain characteristics and are more likely to do better on one treatment than another. Central to this goal is the development of “biomarkers” — biological measures that reflect disease status or effects of treatment — that can flag such patients.
Pharmaceutical and biotechnology companies are especially interested in this approach, as well as studies that can efficiently uncover new biomarkers and subgroups. Statisticians are developing new methods for sifting through vast patient data to identify candidate biomarkers. And they are working on ways to incorporate and reveal biomarkers in the gold standard studies for comparing treatments — randomized clinical trials.
Another perspective focuses on formalizing what clinicians do in practice. For a chronic disease like cancer, doctors make a series of treatment decisions at key disease milestones — such as diagnosis, remission and recurrence. “Personalization” of each decision relies upon doctors synthesizing information on patients using their clinical judgment.
Of keen interest is making this decision-making process “evidence-based” — informed by data. Statisticians are developing new methods for analysis and study design to find data-based sequential decision “rules” that select the best treatment for each patient.
Statistics faculty at NC State are at the forefront of these efforts. With colleagues at UNC-Chapel Hill and Duke, we have a major research project funded by the National Cancer Institute developing new statistical approaches to personalized cancer medicine. And with faculty in NC State’s departments of mathematics and industrial and systems engineering, we have established the faculty cluster on Personalized Medicine Discovery that merges methods across our disciplines toward new data-based approaches.
So the next time you read about a new advance toward personalized medicine, know that statistics undoubtedly played a critical role.
Dr. Marie Davidian is the William Neal Reynolds Professor of Statistics at NC State and the immediate past president of the American Statistical Association (ASA). During her ASA presidency in 2013, she co-curated and contributed to a popular statistics blog at the Huffington Post.
- Categories: